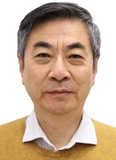
Prof. Jianguo Ma
IEEE Fellow
Zhejiang Lab, China
Biography: Jianguo Ma received the doctoral degree in
engineering in 1996 from
Duisburg University, Duisburg, Germany. He was a faculty member of Nanyang
Technological University (NTU) of Singapore from Sept 1997 to Dec. 2005 after
his post-doctoral fellowship with Dalhousie University of Canada in Apr 1996 –
Sept 1997. He was with the University of Electronic Science and Technology of
China in Jan 2006 – Oct 2009 and he served as the Dean for the School of
Electronic Information Engineering and the founding director of the Qingdao
Institute of Oceanic Engineering of Tianjin University in Oct. 2009 – Aug 2016;
he joined Guangdong University of Technology as a distinguished professor in
Sept 2016 – Aug 2021. Dr. Ma served as the Vice Dean for the School of
Micro-Nano Electronics of Zhejiang University in Sept, 2021 – Oct 2022, Starting
from 1 Nov 2022 he joins the Zhejiang Lab. His research interests are: Microwave
Electronics; RFIC Applications to Wireless Infrastructures; Microwave and THz
Microelectronic Systems;
He served as the Associate Editor for IEEE Microwave and Wireless Components
Letters in 2003 –2005; He was the member for IEEE University Program ad hoc
Committee (2011~2013).
Dr. Ma was the Member of the Editorial Board for Proceedings of IEEE in
2013-2018
He is Fellow of IEEE for the Leadership in Microwave Electronics and RFICs
Applications
Dr. Ma was serving as the Editor-in-Chief of IEEE Transactions on Microwave
Theory and Techniques in 2020 –2022.
Speech Title: AI Empowered Microwave Power-Amplifier Designs
and Optimizations
Abstract: Microwave power amplifiers have been and are still
paying critical
roles in any wireless communication systems and radar systems. The designs of
microwave power-amplifiers are strongly experience-dependent because of the
strong nonlinearities. It is time-consuming and very hard for getting optimal
designs. Conceptual-wise the design procedures of microwave power-amplifiers are
AI algorithms, it is straightforward to make use of AIs as tools for optimizing
power-amplifier designs. The design challenging is discussed firstly followed by
examples of using ANNs. PA-GPT will be the trend.
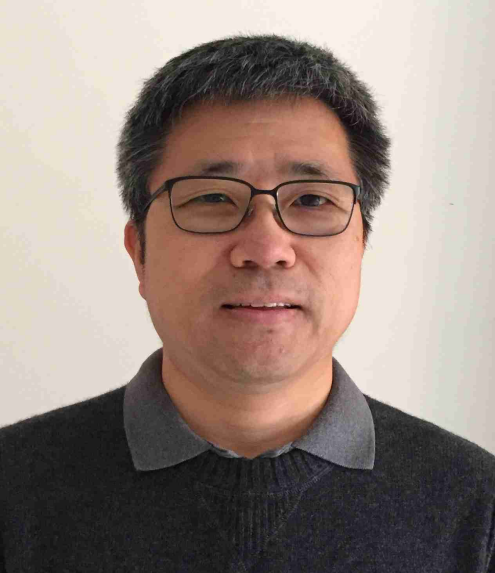
Prof. Bing Liu
AAAI/ACM/IEEE Fellow, University of Illinois at Chicago (UIC), United States
Biography:
Bing Liu is a distinguished professor of Computer Science at the University of
Illinois at Chicago (UIC). He received his Ph.D. in Artificial Intelligence (AI)
from the University of Edinburgh. Before joining UIC, he was a faculty member at
the School of Computing, National University of Singapore (NUS). He was also
with Peking University for one year (2019-2020). His research interests include
lifelong and continual learning, sentiment analysis, lifelong learning chatbots,
open-world AI/learning, natural language processing (NLP), and data mining and
machine learning. He has published extensively in top conferences and journals
(his Google Scholar page). He also authored four books: one about lifelong
machine learning (first ever book on the topic), two about sentiment analysis
and one about Web mining. Three of his papers received Test-of-Time awards: two
from SIGKDD (ACM Special Interest Group on Knowledge Discovery and Data Mining)
and one from WSDM (ACM International Conference on Web Search and Data Mining).
Another of his papers received Test-of-Time award - honorable mention also from
WSDM. Some of his work has been widely reported in the international press,
including a front-page article in the New York Times. On professional services,
he has served as the Chair of ACM SIGKDD from 2013-2017, as program chair of
many leading data mining conferences, including KDD, ICDM, CIKM, WSDM, SDM, and
PAKDD, as associate editor of leading journals such as TKDE, TWEB, DMKD and
TKDD, and as area chair or senior PC member of numerous NLP, AI, Web, and data
mining conferences. He is a recipient of ACM SIGKDD Innovation Award (the most
prestigious technical achievement award from SIGKDD), and he is a Fellow of the
ACM, AAAI, and IEEE.
Title: AI Autonomy: Self‐initiated Open‐world Continual
Learning and Adaptation
Abstract:
As more and more AI agents are used in practice, it is time to think about how
to make these agents fully autonomous so that they can (1) learn by themselves
continually in a self‐motivated and self‐initiated manner rather than being
retrained offline periodically on the initiation of human engineers and (2)
accommodate or adapt to unexpected or novel circumstances. As the real‐world is
an open environment that is full of unknowns or novelties, the capabilities of
detecting novelties, characterizing them, accommodating to them, and gathering
ground‐truth training data and incrementally learning the unknowns/novelties
become critical in making the AI agent more and more knowledgeable, powerful and
self‐sustainable over time.
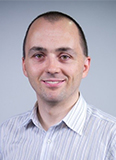
Prof. Philippe Fournier-Viger
Shenzhen University, China
Biography: Philippe Fournier-Viger (Ph.D) is a Canadian researcher,
distinguished professor at Shenzhen University (China). Five years after
completing his Ph.D., he came to China in 2015 and became full professor after
receiving a talent title from the National Science Foundation of China. He has
published more than 375 research papers related to data mining algorithms for
complex data (sequences, graphs), intelligent systems and applications, which
have received more than 13,000 citations. He is the founder of the popular SPMF
data mining library, offering more than 250 algorithms to find patterns in data,
cited in more than 1,000 research papers. He is former associate editor-in-chief
of the Applied Intelligence journal and has been keynote speaker for over 20
international conferences and co-edited four books for Springer. He is a
co-founder of the UDML, PMDB and MLiSE series of workshops held at the ICDM,
PKDD, DASFAA and KDD conferences. Website: http://www.philippe-fournier-viger.com
Speech Title: Advances and challenges for the automatic discovery of interesting
patterns in data
Abstract: Intelligent systems and tools can play an important role in various
domains such as for factory automation, e-business, and manufacturing. To build
intelligent systems and tools, high-quality data is generally required.
Moreover, these systems need to process complex data and can yield large amounts
of data such usage logs, images, videos, and data collected from industrial
sensors. Managing data to gain insights and improve these systems is thus a key
challenge. It is also desirable to be able to extract information or models from
data that are easily understandable by humans. Based on these objectives, this
talk will discuss the use of data mining algorithms for discovering interesting
and useful patterns in data generated from intelligent systems and other
applications.The talk will first briefly review early study on designing
algorithms for identifying frequent patterns. Then, an overview of recent
challenges and advances will be presented to identify other types of interesting
patterns in more complex data. Topics that will be discussed include high
utility patterns, locally interesting patterns, and periodic patterns. Lastly,
the SPMF open-source software will be mentioned and opportunities related to the
combination of pattern mining algorithms with traditional artificial
intelligence techniques for intelligent systems will be discussed.